Machine learning is a vast area and covers several mechanisms of learning such as supervised learning, unsupervised learning, deep learning etc. As a result, there are several frameworks available which include TensorFlow from Google Brain team and Apache MxNet.
Data Science
Data science is also known as data-driven science. It is nothing but a data collected in various forms, either structured or unstructured. Different methods used are machine learning, data mining, analysis of a data, visualization of a data etc.
It is an umbrella that contains many other fields like Machine learning, Data Mining, Big Data, statistics, Data visualization, data analytics etc. It is a “concept to unify statistics, data analysis and their related methods” in order to “understand and analyze actual phenomena” with data.
TensorFlow
As a leading company in Machine Learning, Google had build DistBelief as a proprietary Machine Learning framework. As it’s usage grew, they decided to build the second generation of this library. This also resulted in code refactoring, making it faster and in general elevating the robustness of the application. This new version of Machine Learning framework was named as TensorFlow and was also made “open-source” by Google.
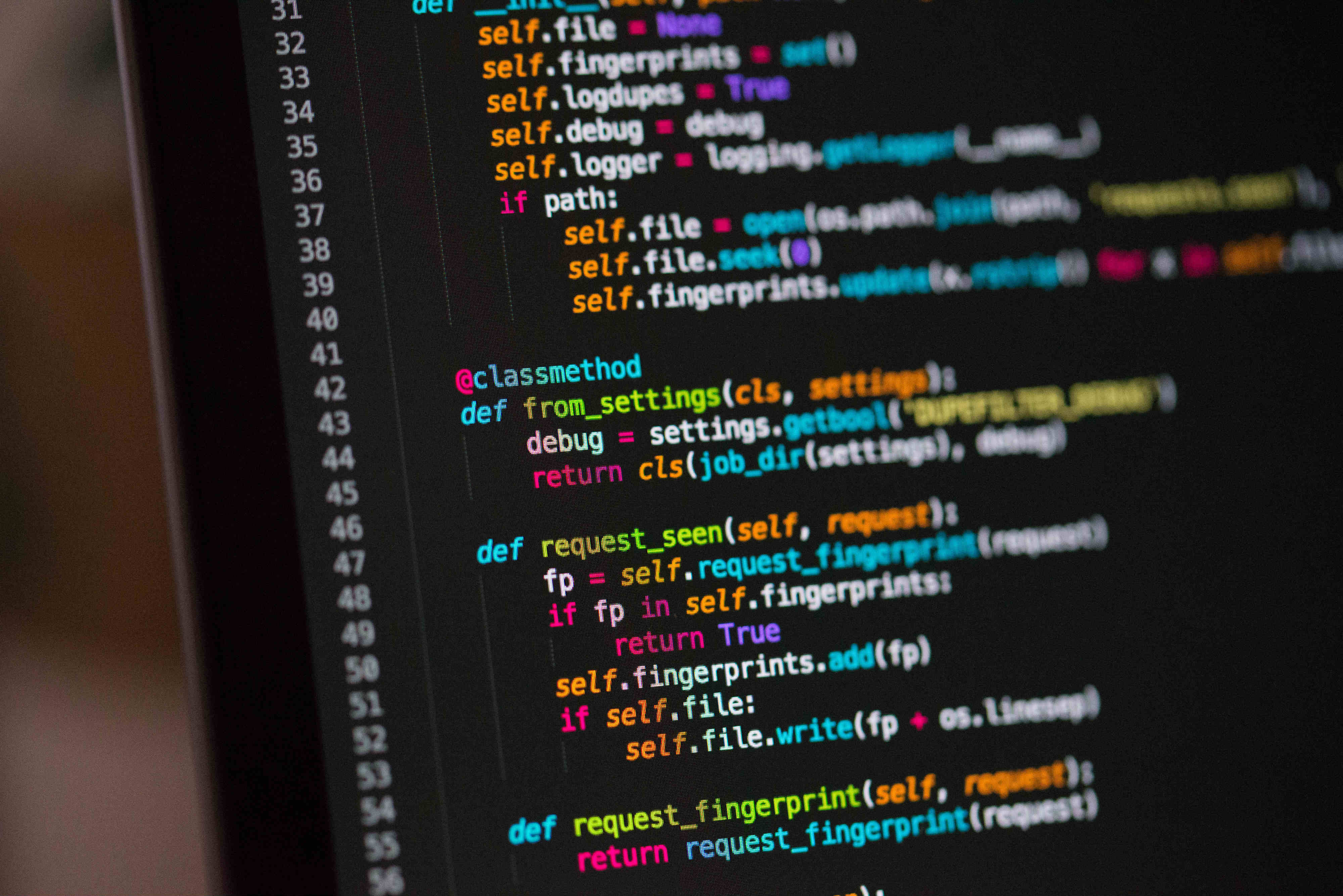
Basics of TensorFlow
Tensor is a multi-dimensional array of base data types. The name TensorFlow is derived from the word “Tensors” and the operations done on the tensors. Machine Learning is all about data and hence tensors are the basis of the framework used for Machine Learning. It is written using C++ and CUDA (Nvidia’s language for programming GPUs). However, it provides APIs for Python, C++, and Java in addition to few others.
TensorFlow allows you to create models for machine learning and also allows you to train those models. Vary famous example usage of TensorFlow is Image recognition. You can use supervised learning method to train the dataset using TensorFlow and then use that model to use it in your application.
While there are some alternatives available, TensorFlow is gaining popularity among the community interested and working in Machine Learning field. It’s APIs are easy to use and allow students and researchers to iterate and create models quickly. It has a high level of accuracy and also has the ability to run on several parallel processors using server farms of GPUs and TPUs (Tensor Processing Units).
Interesting Use cases:
- Image recognition – identify and name objects/persons in a given image
- Text-based applications such as translation and language detection
- Text summarization
- Voice or sound recognition
One of the interesting use cases that I came across was by a company called “Connecterra”. They are using wearable sensors to collect data from cows on farms. Data collected is passed through TensorFlow. A model has been created which allows detecting possible health issues for the cows and giving advanced intimation to farmers. The company is also claiming to show 30% more yield for their customer farmers.
Related Links
- Wikipedia
- TensorFlow Homepage
- TensorFlow Tutorial to get started!
- Alternatives
Related Keywords
Machine Learning, Supervised Learning, GPU, CUDA, Python
Sentiment Analysis
With the increased penetration of internet and mobile, user-generated content is growing at a rapid pace. Also with a cut-throat race to acquire a customer or even to retain a customer, every brand or company needs to understand what their customers are saying. People write their reviews on various websites, facebook, tweet about it or post photos with comments. If brands or companies want to understand if their brand is being talked about in a positive way or a negative way, what they need is to carry out Sentiment Analysis on this data.
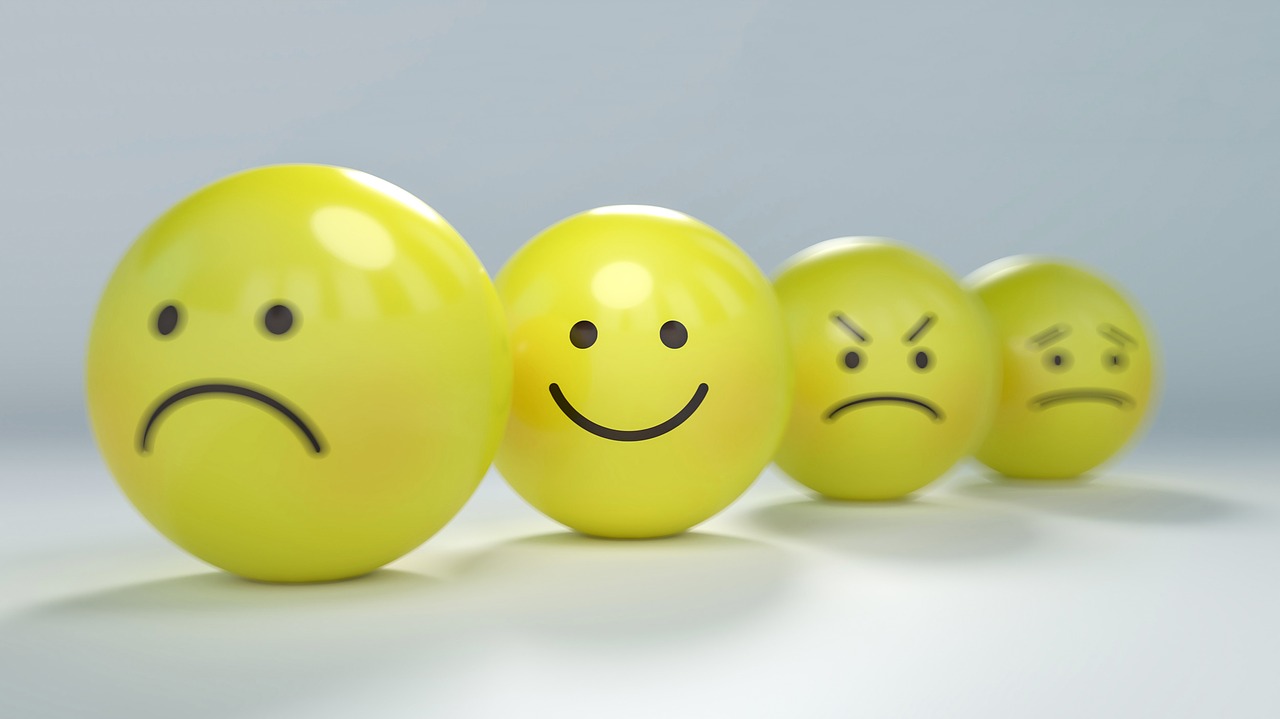
But, what is Sentiment Analysis?
As the name suggests, it is the analysis of data to find out what that data is representing. Are there more happy emotions or sad emotions or there is anger. The Sentiment Analysis tools capture data from various sources. Various types of algorithms are run on this data to identify the emotions appearing in the data. Natural Language Processing (NLP) and Machine Learning (ML) are important backbones of this analysis. NLP allows the tool to process human language. ML allows the tool to learn various moods appearing in the data.
Challenges
Humans have weird ways to express themselves. When someone says “Wow!!”, it could mean real appreciation or it could mean sarcasm. We also say “Hating <brand> is not really my thing” – which may be a positive comment about the brand. Or when we say “He was so aggressive, but then I used to like him” – it represents mixed emotions. “I really love my phone, and I’d hate to lose it” – two different emotions about two different entities. And I can go on and on. Hence the tool has to learn all such variations and then come up with a score which would help the brands and businesses to improve their services. Or such score could also be used to create a marketing plan around the emotions.
The tool analyses words, context, the frequency of words, their occurrences with other words and then starts giving you insights. Take a look at the data gathered from tweets during Chennai Rains in 2015.
You can read the full analysis and see how sentiment analysis could be useful even during crisis situations. Or take a look at data that was analyzed on World Book Day about two biggest e-commerce players (then!!). As you would see, the analysis suggests a marketing plan based on the Sentiment Analysis.
Related Links
Related Keywords
Natural Language Processing (NLP), Machine Learning, Supervised Machine Learning
Supervised Learning
Supervised Learning is a methodology in Machine Learning field. In this methodology, an algorithm is developed based on known dataset and known observations from that dataset. Once the algorithm is stable, researchers / developers use it on new but similar dataset to get the observations about that dataset.
In this method, the known relationship between the dataset (training data) and observations (outcome) helps the algorithm to improve. This is kind of a teacher supervising students in learning new technique. And hence this method is referred as “Supervised Learning”. The developer keeps on improving the algorithm until it reaches fairly accurate outcome for all of the training set.
When to use Supervised Learning?
You have a training data available/gathered. After manual analysis, you know the expected outcome. And then you are required to find out the outcome on another similar dataset for which outcome is not yet available. This is an ideal condition to use Supervised Learning.
Tasks involved in Supervised Learning
Typically there are two types of tasks involved in this type of learning.
- Classification: In this case, the algorithm assigns a category to the input dataset. e.g. If the training dataset is a set of files, this algorithm will categorize each file as text file, image file or binary file.
- Regression: In this task, algorithm will predict a numerical value based on training dataset.
Developers often need to consider “bias vs variance trade-off” while determining the accuracy of the algorithm. Sometimes the algorithm consistently produces incorrect output for given input. This is referred to as “bias”. Sometimes, algorithm produces different values for same input. This is called as “variance”. It is usually impossible to have lowest of both bias as well as variance and hence a balance of these two are required. When such balance is reached, developers can start using that algorithm on different datasets and continue to improve.
Example of Supervised Learning:
Let’s say you have 20 photos and each of them are tagged with labels such as person name, location, type of photo. In this case you will develop a model using this information. Once done, you can feed another 20 photos to this model and see if model has “learnt” from earlier dataset.
Fun Fact:
You can find information on Facebook photos “alt” tag – “Image may contain: mountain, sky, outdoor” OR “Image may contain: One Person, Standing, outdoor” etc. This looks like AI running on the photos through Supervised Learning model.
Reference Links:
Related Keywords:
Machine Learning, Unsupervised Learning, Semi-supervised Learning, Active Learning